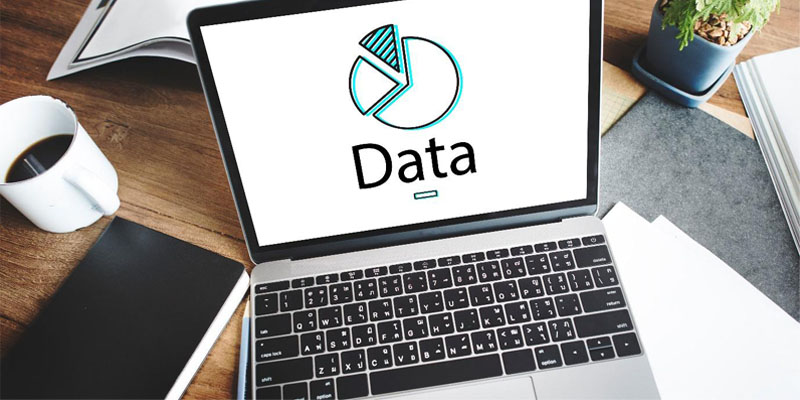
Data cleansing is regarded as the process of correcting data. Particularly, a database may have incomplete, duplicate, or incorrect data that requires fixing. So, this process actually refers to discovering these types of errors and then transforming them into complete and structured niche data. Also called data scrubbing, this process transforms your database into a consistent and reliable source of data to make realistic decisions.
Being an integral part of data management, it combines more subsets that make data ready to use in machine learning and business intelligence. However, the data flows all around through smart devices, but that is not all useful. Any entrepreneur or organization requires valuable and precise datasets to make strategies and informed decisions. Here, data scientists and business intelligence experts appear in a pivotal role.
Why data cleaning?
A business is all about taking risks and making decisions. Data makes it way easier to make those decisions. But if your data is unclean, the resulting decisions would lead to unreal results. In the present scenario, organizations rely on data analytics, for which data is a must. So, the decision can be related to operations, teams, marketing, sales, or anything. It requires a source of clean and valid information. This is where data cleansing solutions emerges as a key to unlocking realistic decisions.
Just think of a scenario wherein the source details are incomplete. They are full of mistakes, and understanding them is no less than a herculean task. Imagine how flawed your decisions would be. Certainly, your data-driven strategies won’t be as effective as they could be with clean data. Faulty data would lead to bad decisions, which may push you to suffer many missed opportunities and challenges. This would definitely put a burden on your budget, as the operational costs would be higher than the revenue and profits. This is something you should brainstorm.
What Types of Errors Can Data Cleansing Fix?
Well, data cleansing fixes data by removing errors. It addresses a series of errors like inaccuracy, invalid data, incompatibility, and corrupt entries. The causes of these problems can be manual data handling, management, or data entry. Besides, data migration, restructuring, formatting, and other reasons can be the reasons behind it.
So here, some common errors are mentioned to take note of and be attentive to.
- Typos and invalid or missing data
Typos are typing errors. And invalid data refers to datasets that are obsolete. And missing entries are simply such datasets that are required but are not there. Simply put, spelling mistakes, wrong numerals or syntax, and missing values can be a matter of concern.
- Inconsistent data
Inconsistent data refers to erroneous names, addresses, and other attributes connected to datasets. These can be related to missing surnames, middle names, or zip codes in a series of data points. Overall, the cleansing process proves a milestone in establishing consistency among datasets.
- Duplicate data
This is related to duplications or entries that are the same. This process can help identify those duplicates and make the entire database error-free. Certainly, it may require a data analyst to use validation techniques like Vlookup and the best data quality management practices. The main reason for the emergence of dupes is data migration or mergers. So, always follow these processes with care.
- Irrelevant data
The next is related to out-of-data entries. These entries can be called outliers, which means that they don’t belong to the database where they are. They may be correct but not relevant. So, this process guides the removal of useless entries and prepares the whole database for analysis. It cuts short the process of data processing and also saves storage.
Steps of the Data Cleansing Process
In every operation where records are maintained, data cleansing plays a pivotal role. It certainly guides in making such decisions that are realistically achievable. Considering the vitality of this process, the following steps are given to acknowledge it:
- Inspection and profiling
The very first step is collecting and auditing the quality of the data to see if there are any errors. It starts with the profiling of datasets, which shows relational datasets or elements. It means that likewise, datasets are kept together. It eases their quality management and analysis. Some skilled data analysts find errors effortlessly. This is the biggest benefit of profiling.
- Cleaning or scrubbing
This is obviously related to fixing all errors like duplication, missing details, inconsistency, formatting errors, redundant entries, or typos.
- Verifying Data
Once the entire database is cleaned, quality analysts come into the spotlight. They filter erroneous data and verify if all sets are error-free. Overall, they conform if the details correspond to quality rules and standards.
- Reporting
Once the cleaning is over, the optimized entries are recorded in a report. It also consists of data quality trends and progress that should be recognized. Additionally, all issues that they found and have corrected are highlighted to further keep them in mind while processing.
These common steps help data analysts move towards data preparation for analysis, which starts with structuring and data transformation.
The Benefits of Clean Data
Considering the business aspect, clean information proves a difference-maker. Here are some specific ones.
- Improved decision-making
Providing accurate data for analysis leads to accurate decisions and productive results, which prove effective. This enables any organization to make data-driven decisions or outline strategies for effective business management and backend operations.
- More effective marketing and sales
With all the clean details regarding your customers, their behavior, and the market, it’s easy to make decisions that make customers happy. This is how you can establish a strong sales system and customer relationship management that have strategies, offers, and programs integrated for customers.
- Better operational performance.
Maintaining updated and clean data can show you prospective challenges, shortages in stock or inventories, and gaps in deliveries. Also, bottlenecks will be crystal clear, which may be putting an extra burden on your shoulders and resulting in low revenues and a bad customer experience.
- Increased use of data
In addition to the aforementioned benefits, clean details can prove their true value. You can use it to understand the genuine problems of customers or defects in your products or services. This knowledge can guide you to add a little more value, innovate, and serve. This will help in enhancing customer relationships and building trust.
- Reduced data costs
What if you are likely to run out of a product in your inventory and it is in high demand? Certainly, this will be a big loss. The data that is up-to-date can help inform us about prospects, gaps, or challenges. And this information can multiply your revenue while saving time because you would make an informed decision to increase the quantity of the most demanded product.
Besides, this practice can also help improve data governance.
Conclusion
Data cleansing is the process of scrubbing the details that you have and keeping them for decision-making. Certainly, it empowers any business to use it for making profitable strategies that can multiply revenues. It benefits businesses by providing prestigious data that produces realistic decisions.